Heisenberg Professorship for Carsten Schilde Battery research: How artificial intelligence can advance process engineering
As part of the Heisenberg Professorship on “Digitale Methoden für komplexe Systeme in der Verfahrens- und Fertigungstechnik“ (Digital Methods for Complex Systems in Process and Production Engineering), which was awarded to Professor Carsten Schilde in 2022, researchers are working on methods of artificial intelligence (AI) in addition to classical simulation methods. With their help, the understanding and control of process engineering processes along the manufacturing process chain should be facilitated in the future. This knowledge can also play an important role in battery research.
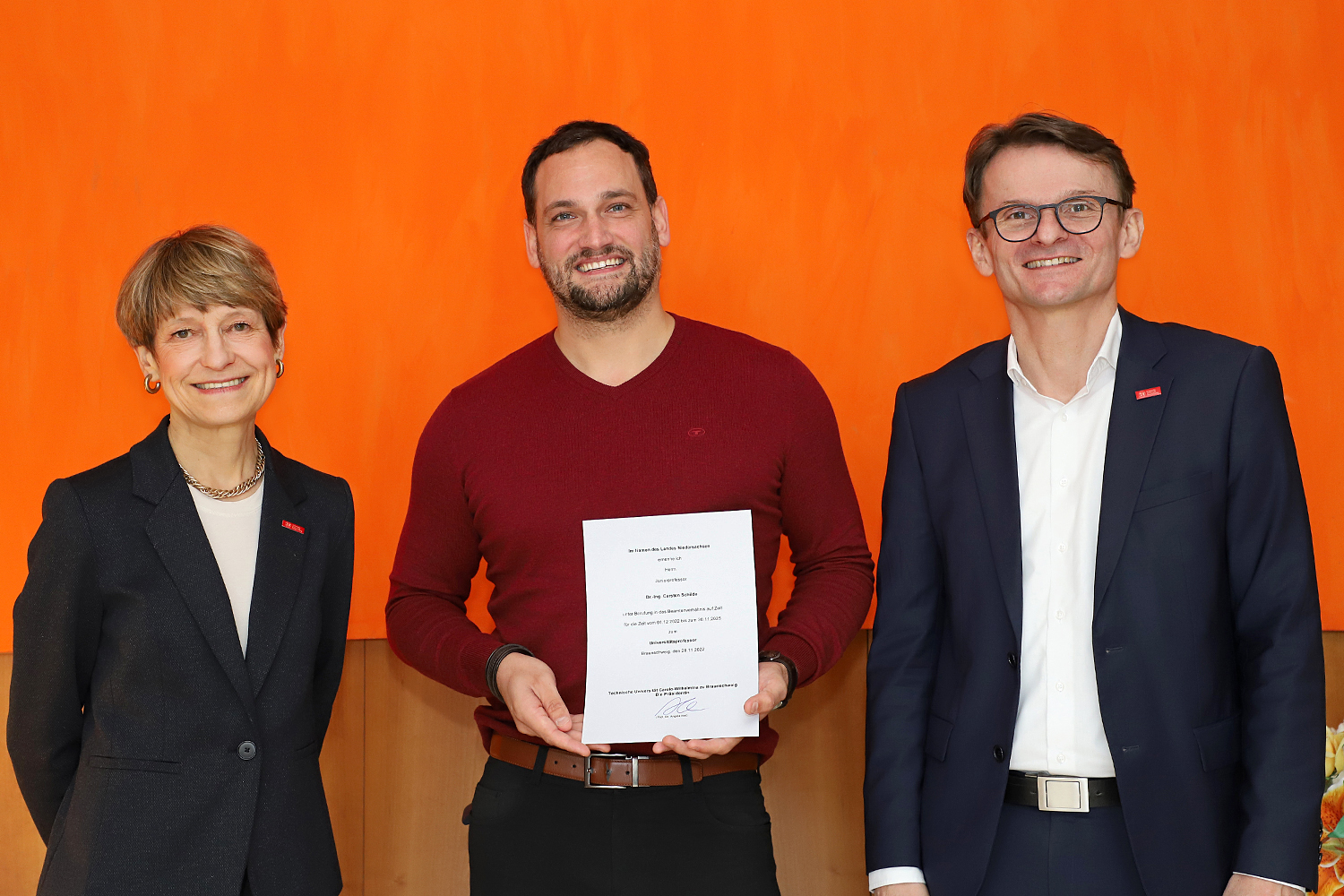
Professor Carsten Schilde with President Angela Ittel and Professor Klaus Dröder (Dean of the Faculty of Mechanical Engineering). Photo credit: Kristina Rottig/TU Braunschweig
The potential applications of AI methods in this field are not only diverse, but also hold a lot of untapped potential, e.g. in predictive modelling, process and formulation optimisation, error detection, uncertainty assessment, intelligent process control, scale transfer and mechanistic modelling.
In recent years, the working group has increasingly focused on methods of artificial intelligence (genetic and symbolic programming, genetic algorithms, various neural networks, etc.) in connection with experiment and numerical simulation. Among other things, the focus is on hybrid modelling approaches via genetic and symbolic programming, the development of an ML Expert management system as well as projects on ML-based machine control, DEM-ML surrogate models or the programming of new AI methods based on combinatorics.
For example, the working group is working on a number of projects with the aim of deriving data-based physically inspired hybrid models that describe, among other things, the complex processes involved in the production of battery electrodes. These models are derived by a hybrid modelling approach developed at the Institute for Particle Technology (iPAT) and could play a decisive role in the design, optimisation and transfer of materials and processes along the entire value chain.
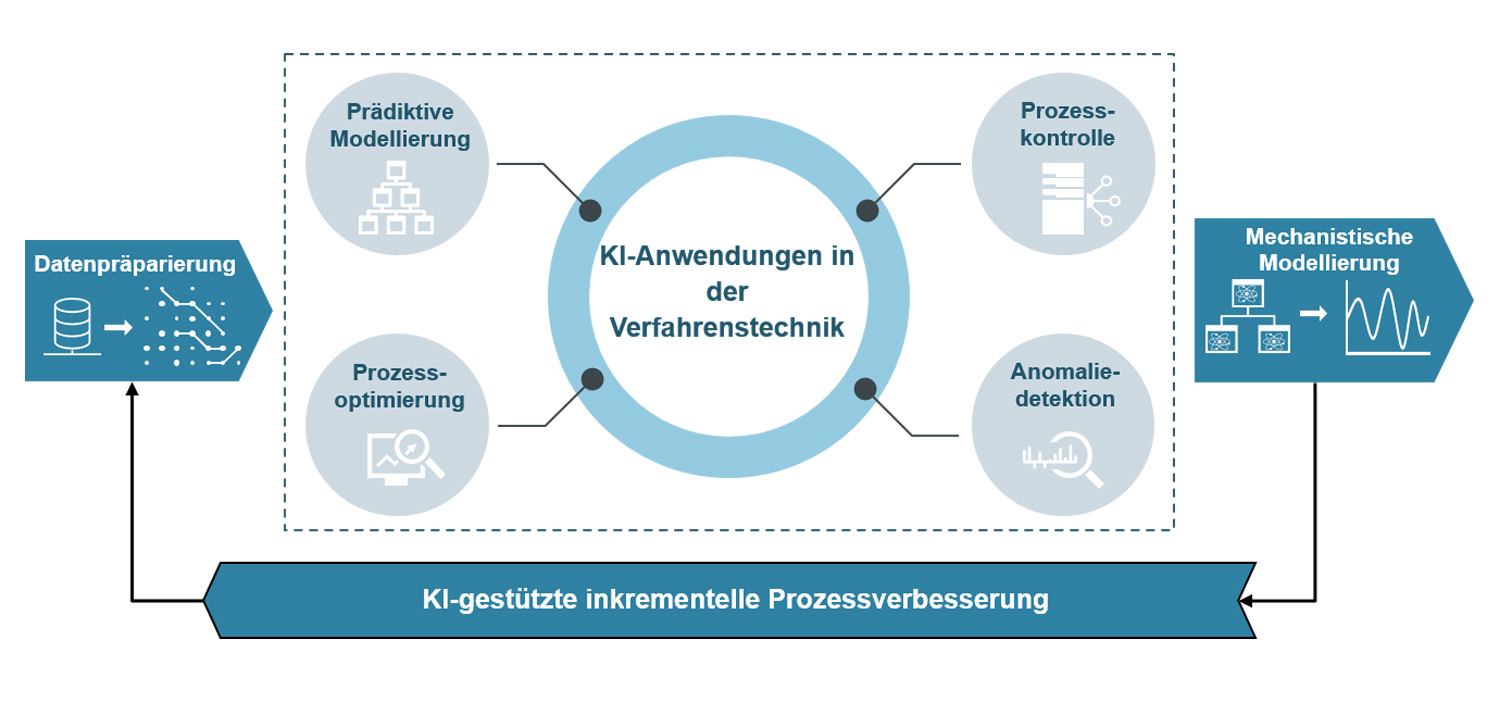
Illustration of AI applications in process engineering. Picture credit: Ahmed Eisa/TU Braunschweig
In order to generate suitable data for hybrid modelling, data acquisition systems are being further integrated into existing processes in cooperation with the Battery Process Technology research area at iPAT. In the future, these models will be used as reverse engineering strategies in order to gain an even deeper understanding of the investigated product development processes and thus enable the sustainable development of Germany as a technology location and secure global competitiveness in battery process technology.