Consistency for image recognition of vehicles Best Paper Award goes to the Institute for Communications Technology for the third time in a row
The CVPR (Conference on Computer Vision and Pattern Recognition) is the most important conference in the field of machine vision. With Professor Tim Fingscheidt, Serin Varghese, Andreas Bär and Marvin Klingner, scientists from TU Braunschweig won a Best Paper Award at one of the conference workshops for the third time in a row. Together with Volkswagen Group Automation, the researchers from the Institute for Communications Technology developed a training system that enables vehicles to assess their surroundings more safely.
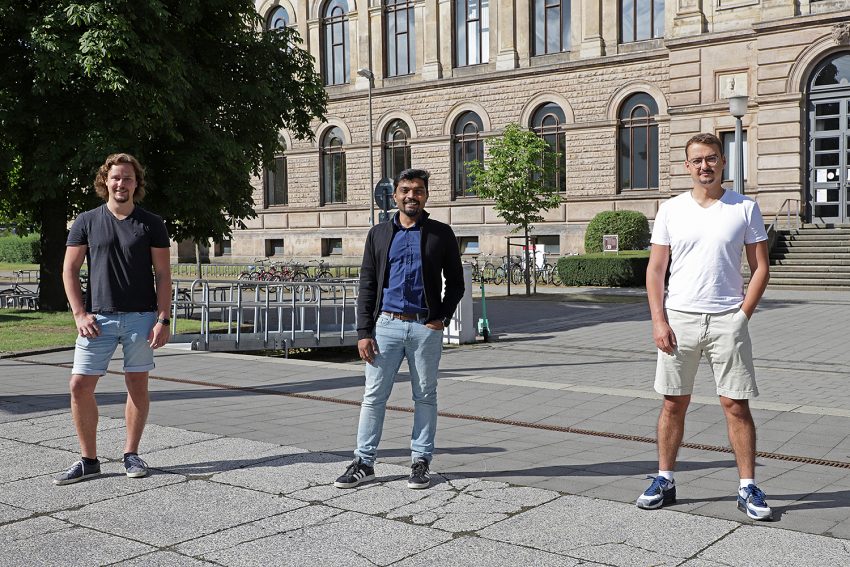
The three award-winning doctoral students from the Institute for Communications Technology: (from left) Marvin Klingner, Serin Varghese and Andreas Bär. Photo credit: Kristina Rottig/TU Braunschweig.
The workshop was about the safe use of artificial intelligence for autonomous driving. Artificial neural networks primarily process the images that autonomous vehicles take of their surroundings. They use data to automatically learn what surrounds them and how far away it is. The central method for environment perception is semantic segmentation. Here, the neural networks classify each pixel into road, car or passer-by in a fraction of a second.
- Photo credit: The Cityscapes Dataset, IfN/TU Braunschweig
- Photo credit: The Cityscapes Dataset, IfN/TU Braunschweig
In principle, the performance of artificial intelligence in semantic segmentation is very fast and reliable. However, in the case of a video, i.e. a rapid sequence of many individual images, contradictions can occur. “For example, a cyclist can be recognised in one image and completely overlooked in the next,” explains Marvin Klingner, one of the authors. “When the computer then combines the information, it has to make a clear decision from the contradictory information. The question of which information is the correct one is particularly difficult for the computer.”
With the right training to consistency
The scientists solved the problem by forcing the neural network to be consistent over time during the learning process. As a rule, one trains here image by image and optimises the neural network in such a way that it delivers the best possible results on each individual image. In addition, the scientists used image sequences. In doing so, they sanctioned if the neural network was inconsistent in the description of the sequences. In this way, the network learns to provide temporally consistent descriptions and continuously improves in the course of the training.
Serin Varghese, also one of the authors: “This kind of learning process is not completely new in itself. But normally the artificial intelligence would then also need videos in the input, which is very computationally expensive. The special case in our paper: you only need the videos for training and in the application it still works with single images.”