Recognising the Unknown Review of the Deep Learning Lab 2023
“What can be seen in this picture?” What is a simple question for us humans is a tricky matter for machines. Often they can only classify living beings or objects in pictures into a limited number of different classes. But what if what they see via their cameras, for example, cannot be classified into these classes? This question was investigated by 30 students in this year’s Deep Learning Lab and they developed solutions for so-called “anomaly segmentation”.
Person or car? Machines, such as autonomous vehicles, can distinguish this relatively easily. The AI segments the image that it perceives via its cameras. Pixel by pixel, it assigns familiar classes such as “person” or “car”. Such segmentation is trained by showing the neural network a large number of examples of images with the corresponding “Ground Truth”. The “Ground Truth” are images that have been divided into classes by humans in advance and serve as training data. Since the network is trained with this training data, it only knows a certain and above all limited number of typical or also “normal” classes that are contained in the training data. However, the number of possible object classes in the world is not limited. Thus it happens that the network does not recognise living beings or objects because they are unknown to it. “In the application case, this is a risk because potential dangerous situations are not recognised in this way,” explains Jasmin Breitenstein, research associate at the Institute for Communications Technology and member of the Deep Learning Lab’s organisational team.
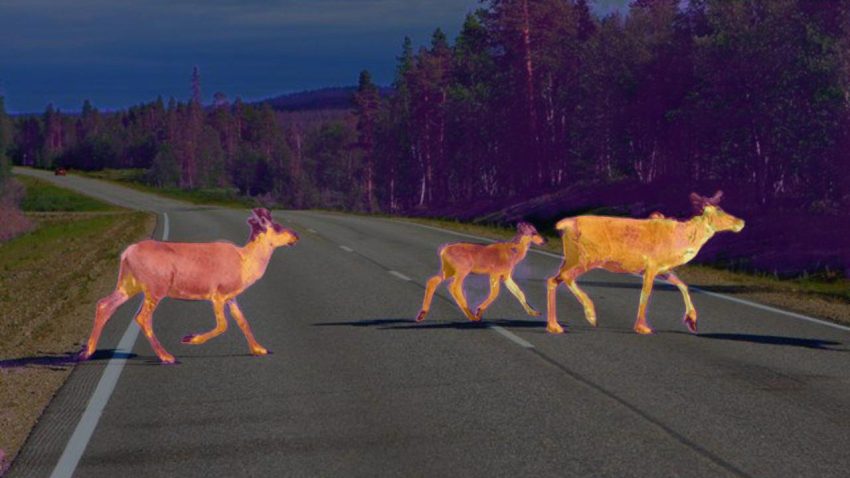
An anomaly segmentation as performed by the neural network. The hinds are unknown to the AI and are perceived as an anomaly. Photo credit: SegmentMeIfYouCan Dataset, IfN/TU Braunschweig
Training machines to classify unknowns correctly
Finding solutions for recognising unknown objects (=anomalies) was the task of the students in the Machine Learning Challenge of the Deep Learning Lab this year. The participating students received a comparison method and had to improve it in teams of three or implement their own approach to segment anomalies. The submitted methods were then evaluated on a test data set unknown to the participants. In this evaluation, metrics were measured that indicate how well the anomalies present in the test data set were segmented.
In addition to the prizes for the best solution, an environmental prize was also awarded for the team that achieved very good results with the least computing time, and accordingly also with the lowest electricity consumption. The prizes were 150 euros per person for first place, 100 euros for second place, 50 euros for third place and another 150 euros for the environmental prize.
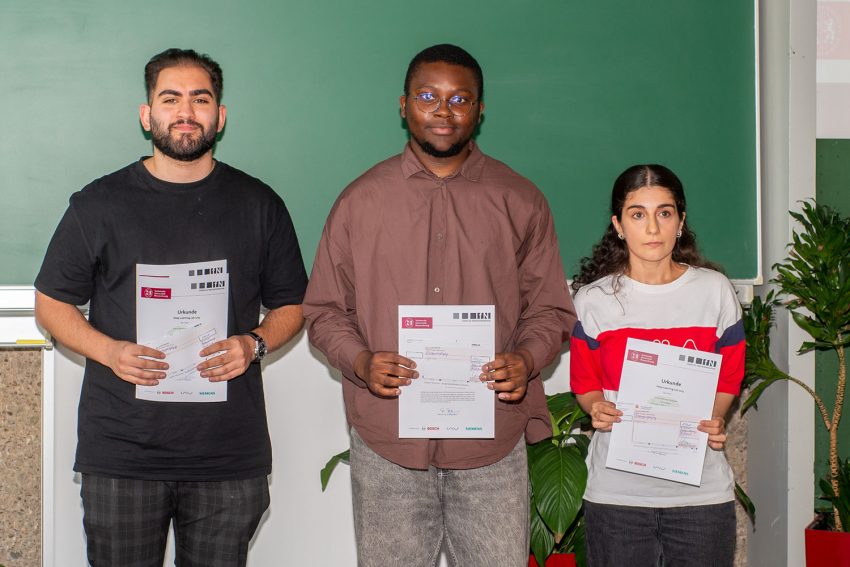
The winning team of the Machine Learning Challenge 2023 around Emre Gezer, Emmanuel-Wilson Bauni Kamga and Amira Mahmod Darwisch (from left to right). Photo credit: Andreas Gudat/TU Braunschweig
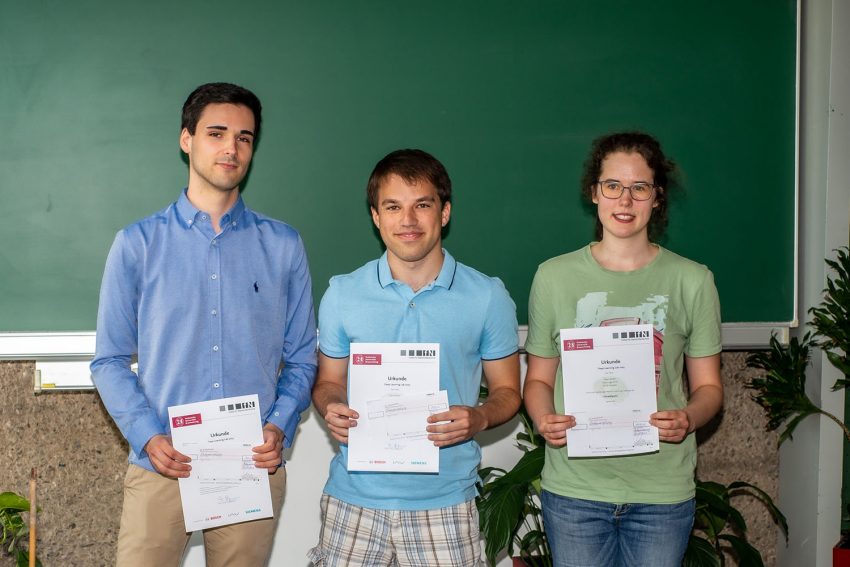
The environmental prize and second place this year went to Fabian Bettels, Haljan Lugo Girao and Svenja Lamottke (from left to right). Photo credit: Andreas Gudat/TU Braunschweig
This year’s winner was the group consisting of Amira Mahmod Darwisch, Emre Gezer and Emmanuel-Wilson Bauni Kamga. Second place went to Fabian Bettels, Haljan Lugo Girao and Svenja Lamottke. The 3rd place went to Jonas Busse, Zhengduo Song and Angelo Steinmetzer. The environmental prize was awarded to Fabian Bettels, Haljan Lugo Girao and Svenja Lamottke, who achieved very good results with only 411 hours of computing time on the working group’s GPU cluster. They thus best achieved the balance between little computing time (i.e. low electricity consumption) and good performance of the anomaly segmentation.
Great popularity with students and sponsors
The Deep Learning Lab is a machine learning practical training for Master’s students and has been taking place since 2018. Until 2019, the DLL was funded as a project by the Federal Ministry of Education and Research (BMBF). In addition, several companies support the Deep Learning Lab and donate the prize money. The award ceremony also included some networking opportunities for the students to establish contacts between companies and students.
As in previous years, the organisers were able to fill the 30 places for the Deep Learnings Lab from numerous applications. Participants and organisers could also count on the support of the sponsors again this year. “In my 17 years at TU Braunschweig, the Deep Learning Lab has been the most successful teaching project. The enormous broad impact on students and sponsors can also be seen in the fact that some of those who support the Lab today as representatives of the sponsors took part in the Deep Learning Lab themselves as students a few years ago,” says Prof. Tim Fingscheidt, the initiator of the Deep Learning Lab.